Inside Amazon's Race to Build the AI Industry's Biggest Datacenters

Rami Sinno is crouched beside a filing cabinet, wrestling a beach-ball sized disc out of a box, when a dull thump echoes around his laboratory.
“I just dropped tens of thousands of dollars’ worth of material,” he says with a laugh.
Straightening up, Sinno reveals the goods: a golden silicon wafer, which glitters in the fluorescent light of the lab. This circular platter is divided into some 100 rectangular tiles, each of which contains billions of microscopic electrical switches. These are the brains of Amazon’s most advanced chip yet: the Trainium 2, announced in December.
For years, artificial intelligence firms have been dependent on one company, Nvidia, to design the cutting-edge chips required to train the world’s most powerful AI models. But as the AI race heats up, cloud giants like Amazon and Google have accelerated their in-house efforts to design their own chips, in pursuit of market share in the rapidly-growing cloud computing industry, which was valued at $900 billion at the beginning of 2025.
This unassuming Austin, Texas, laboratory is where Amazon is mounting its bid for semiconductor supremacy. Sinno is a key player. He’s the director of engineering at Annapurna Labs, the chip design subsidiary of Amazon’s cloud computing arm, Amazon Web Services (AWS). After donning ear protection and swiping his card to enter a secure room, Sinno proudly displays a set of finished Trainium 2s, which he helped design, operating the way they normally would in a datacenter. He must shout to be heard over the cacophony of whirring fans that whisk hot air, warmed by these chips’ insatiable demand for energy, into the building’s air conditioning system. Each chip can fit easily into the palm of Sinno’s hand, but the computational infrastructure that surrounds them—motherboards, memory, data cables, fans, heatsinks, transistors, power-supplies—means this rack of just 64 chips towers over him, drowning out his voice.
Large as this unit may be, it’s only a miniaturized simulacrum of the chips’ natural habitat. Soon thousands of these fridge-sized supercomputers will be wheeled into several undisclosed locations in the U.S. and connected together to form “Project Rainier”—one of the largest datacenter clusters ever built anywhere in the world, named after the giant mountain that looms over Amazon’s Seattle headquarters.
Project Rainier is Amazon’s answer to OpenAI and Microsoft’s $100 billion “Stargate” project, announced by President Trump at the White House in January. Meta and Google are also currently building similar so-called “hyperscaler” datacenters, costing tens of billions of dollars apiece, to train their next generation of powerful AI models. Big tech companies have spent the last decade amassing huge piles of cash; now they’re all spending it in a race to build the gargantuan physical infrastructure necessary to create AI systems that, they believe, will fundamentally change the world. Computational infrastructure of this scale has never been seen before in human history.
The precise number of chips involved in Project Rainier, the total cost of its datacenters, and their locations are all closely-held secrets. (Although Amazon won’t comment on the cost of Rainier by itself, the company has indicated it expects to invest some $100 billion in 2025, with the majority going toward AWS.) The sense of competition is fierce. Amazon claims the finished Project Rainier will be “the world’s largest AI compute cluster”—bigger, the implication is, than even Stargate. Employees here resort to fighting talk in response to questions about the challenge from the likes of OpenAI. “Stargate is easy to announce,” says Gadi Hutt, Annapurna’s director of product. “Let’s see it implemented first.”
Amazon is building Project Rainier specifically for one client: the AI company Anthropic, which has agreed to a long lease on the massive datacenters. (How long? That’s classified, too.) There, on hundreds of thousands of Trainium 2 chips, Anthropic plans to train the successors to its popular Claude family of AI models. The chips inside Rainier will collectively be five times more powerful than the systems that were used to the best of those models. “It’s way, way, way bigger,” Tom Brown, an Anthropic co-founder, tells TIME.
Nobody knows what the results of that huge jump in computational firepower will be. Anthropic CEO Dario Amodei has publicly predicted that “powerful AI” (the term he prefers over Artificial General Intelligence—a technology that can perform most tasks better and more quickly than human experts) could arrive as early as 2026. That means Anthropic believes there’s a strong possibility that Project Rainier, or one of its competitors, will be the place where AGI is birthed.
The flywheel effect
Anthropic isn’t just a customer of Amazon; it’s also partially owned by the tech giant. Amazon has invested $8 billion in Anthropic for a minority stake in the company. Much of that money, in a weirdly circular way, will end up being spent on AWS datacenter rental costs. This strange relationship reveals an interesting facet of the forces driving the AI industry: Amazon is essentially using Anthropic as a proof-of-concept for its AI datacenter business.
It’s a similar dynamic to Microsoft’s relationship with OpenAI and Google’s relationship with its DeepMind subsidiary. “Having a frontier lab on your cloud is a way to make your cloud better,” says Brown, the Anthropic co-founder who manages the company’s relationship with Amazon. He compares it to AWS’s partnership with Netflix: in the early 2010s, the streamer was one of the first big AWS customers. Because of the huge infrastructural challenge of delivering fast video to users all over the world, “it meant that AWS got all the feedback that they needed in order to make all of the different systems work at that scale,” Brown says. “They paved the way for the whole cloud industry.”
All cloud providers are now trying to replicate that pattern in the AI era, Brown says. “They want someone who will go through the jungle and use a machete to chop a path, because nobody has been down that path before. But once you do it, there’s a nice path, and everyone can follow you.” By investing in Anthropic, which then spends most of that money on AWS, Amazon creates what it likes to call a flywheel: a self-reinforcing process that helps it build more advanced chips and datacenters, drives down the cost of the “compute” required to run AI systems, and shows other companies the benefits of AI, which in turn results in more customers for AWS in the long run. Startups like OpenAI and Anthropic get the glory, but the real winners are the big tech companies who run the world’s major cloud platforms.
To be sure, Amazon is still heavily reliant on Nvidia chips. Meanwhile, Google’s custom chips, known as TPUs, are considered by many in the industry to be superior to Amazon’s. And Amazon isn’t the only big tech company with a stake in Anthropic. Google has also invested some $3 billion for a 14% stake. Anthropic uses both Google and Amazon clouds in a bid to be reliant on neither. Despite all this, Project Rainier and the Trainium 2 chips that will fill its datacenters are the culmination of Amazon’s effort to accelerate its flywheel into pole position.
Trainium 2 chips, Sinno says, were designed with the help of intense feedback from Anthropic, which shared details with AWS about how its software interacted with Trainium 1 hardware, and made suggestions for how the next generation of chips could be improved. Such tight collaboration isn’t typical for AWS clients, Sinno says, but is necessary for Anthropic to compete in the cutthroat world of “frontier” AI. The capabilities of a model are essentially correlated with the amount of compute spent to train and run it, so the more compute you can get for your buck, the better your final AI will be. “At the scale that they’re running, each point of a percent improvement in performance is of huge value,” Sinno says of Anthropic. “The better they can utilize the infrastructure, the better the return on investment for them is, as a customer.”
The more sophisticated Amazon’s in-house chips become, the less it will need to rely on industry leader Nvidia—demand for whose chips far outstrips supply, meaning Nvidia can pick and choose its customers while charging well above production costs. But there’s another dynamic at play, too, that Annapurna employees hope might give Amazon a long-term structural advantage. Nvidia sells physical chips (known as GPUs) directly to customers, meaning that each GPU has to be optimized to run on its own. Amazon, meanwhile, doesn’t sell its Trainium chips. It simply sells access to them, running in AWS-operated datacenters. This means Amazon can find efficiencies that Nvidia would find difficult to replicate. “We have many more degrees of freedom,” Hutt says.
Back in the lab, Sinno returns the silicon wafer to its box and moves to another part of the room, gesturing at the various stages of the design process for chips that might—potentially very soon—help summon powerful new AIs into existence. He is excitedly reeling off statistics about the Trainium 3, expected later this year, which he says will be twice the speed and 40% more energy-efficient than its predecessor. Neural networks running on Trainium 2s assisted with the team’s design of the upcoming chip, he says. That’s an indication of how AI is already accelerating the speed of its own development, in a process that is getting faster and faster. “It’s a flywheel,” Sinno says. “Absolutely.”
Trump tariffs impact supply chains Apple developed to reduce its China dependency
Apple has warned that tariffs could hurt its business, prompt it to increase prices and potentially force it to stop offering certain products altogether.
The Federal Reserve is not likely to rescue markets and economy from tariff turmoil anytime soon
Should the president hold fast to his tougher-than-expected trade policy, there's a material risk of at least near-term costs.
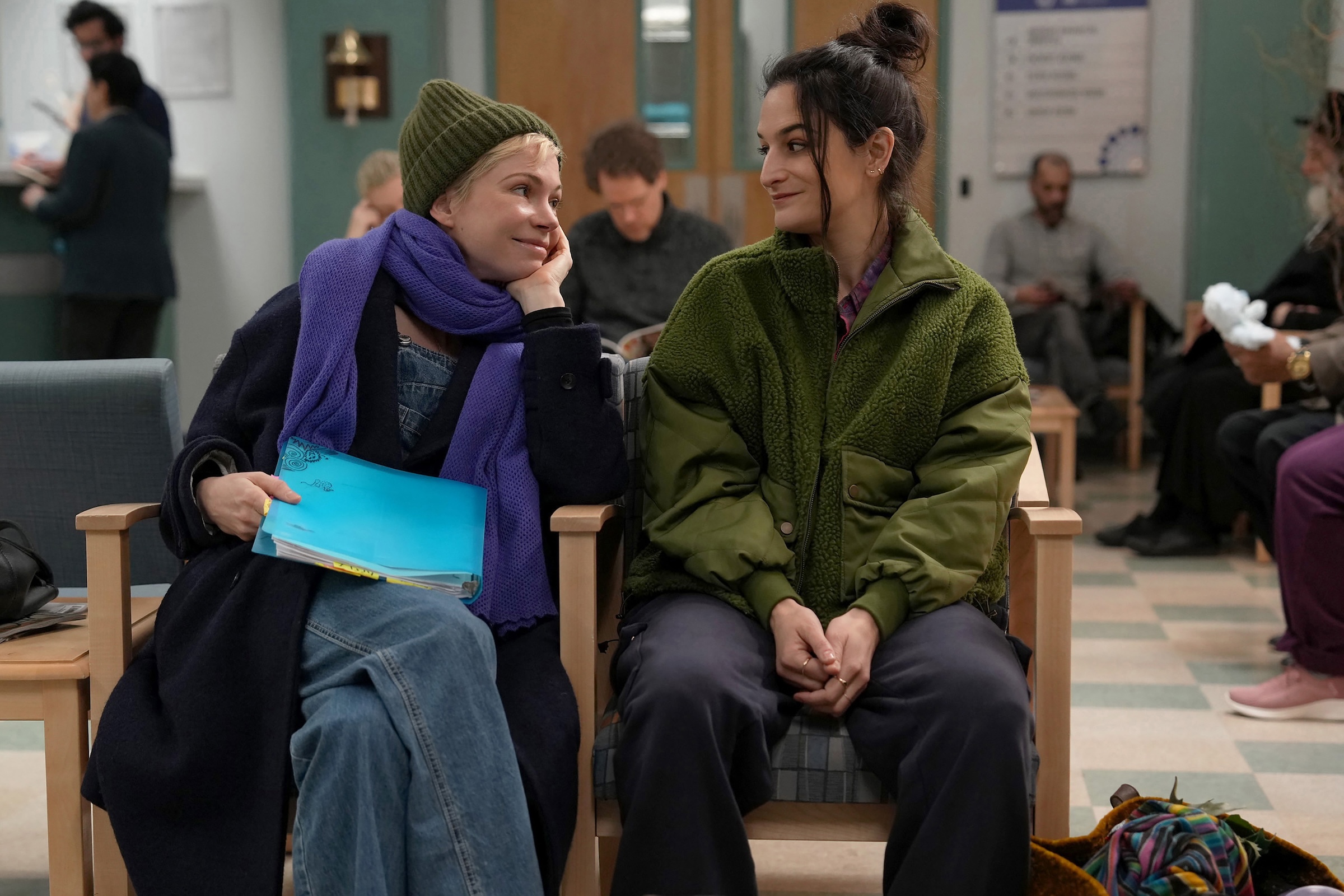
The Radical and Moving True Story Behind FX's Dying for Sex
Bitcoin falls to $81,000, crypto stocks tank as Trump tariffs rock markets: CNBC Crypto World
On today's show, major cryptocurrencies and crypto-focused stocks were under pressure after President Trump's big tariffs reveal jolted the stock market. Plus, the House Financial Services Committee voted in favor the STABLE Act, a bill aimed at creating a regulatory framework for stablecoins. And, Stuart Alderoty, chief legal officer at Ripple, discusses the findings of a survey conducted by a crypto-focused nonprofit the company helped support called the National Cryptocurrency Association.
Pentagon watchdog launches probe of Defense Secretary Pete Hegseth over use of Signal app
The use of the Signal messaging app by top Trump officials came to light after The Atlantic's editor was added to a group chat about planned Houthi strikes.